The best solutions are far more effective than others
Scared Straight was a government programme that received billions of dollars of funding, and was profiled in an award-winning documentary. The idea was to take kids who committed crimes, show them life in jail, and scare them into embracing the straight and narrow.
The only problem? One meta-analysis found the programme made the kids more likely to commit crimes, and another more recent meta-analysis found it had no effect.1
Causing this much harm is rare, but when social programmes are rigorously tested, a large fraction of them don’t work.2
So, even if you’ve chosen a pressing issue, it would be easy to end up working on a solution to it that has very little impact.
Meanwhile, research finds that among solutions that do have positive effects, the best interventions within an area are far more cost effective than average — often achieving over 10 and sometimes 100 times as much for a given unit of resources.
Taking a career that lets you work on more effective solutions is one way to find a greater opportunity to contribute.
In this article, we explain what we think the current research implies about how much solutions differ in effectiveness, why this should change how we approach making a difference, and how to find the best solutions within an area in practice.
Table of Contents
How much do solutions differ in how well they work?
In recent years there’s been a wave of advocacy to stop the use of plastic bags. However, convincing someone to entirely give up plastic bags for the rest of their life (about 10,000 bags) would avoid about 0.1 tonnes of CO2 emissions. In contrast, convincing someone to take just one fewer transatlantic flight would reduce CO2 emissions by over one tonne — more than 10 times as much.3
And rather than trying to change personal consumption in the first place, we’d argue you could do even more to reduce emissions by advocating for greater funding of neglected green technology.
This pattern doesn’t just hold within climate change. Its significance was first pointed out in the field of global health, by Toby Ord’s article “The Moral Imperative toward Cost-Effectiveness in Global Health.”
He found data that compared different health interventions in poor countries (e.g. malaria bed nets, vaccines, types of surgery) in terms of how many years of healthy life they produce per $1,000 invested.
This data showed that the most cost-effective interventions were around 50 times as cost effective as the median, 23 times the mean, and almost exactly obeyed the 80/20 rule.
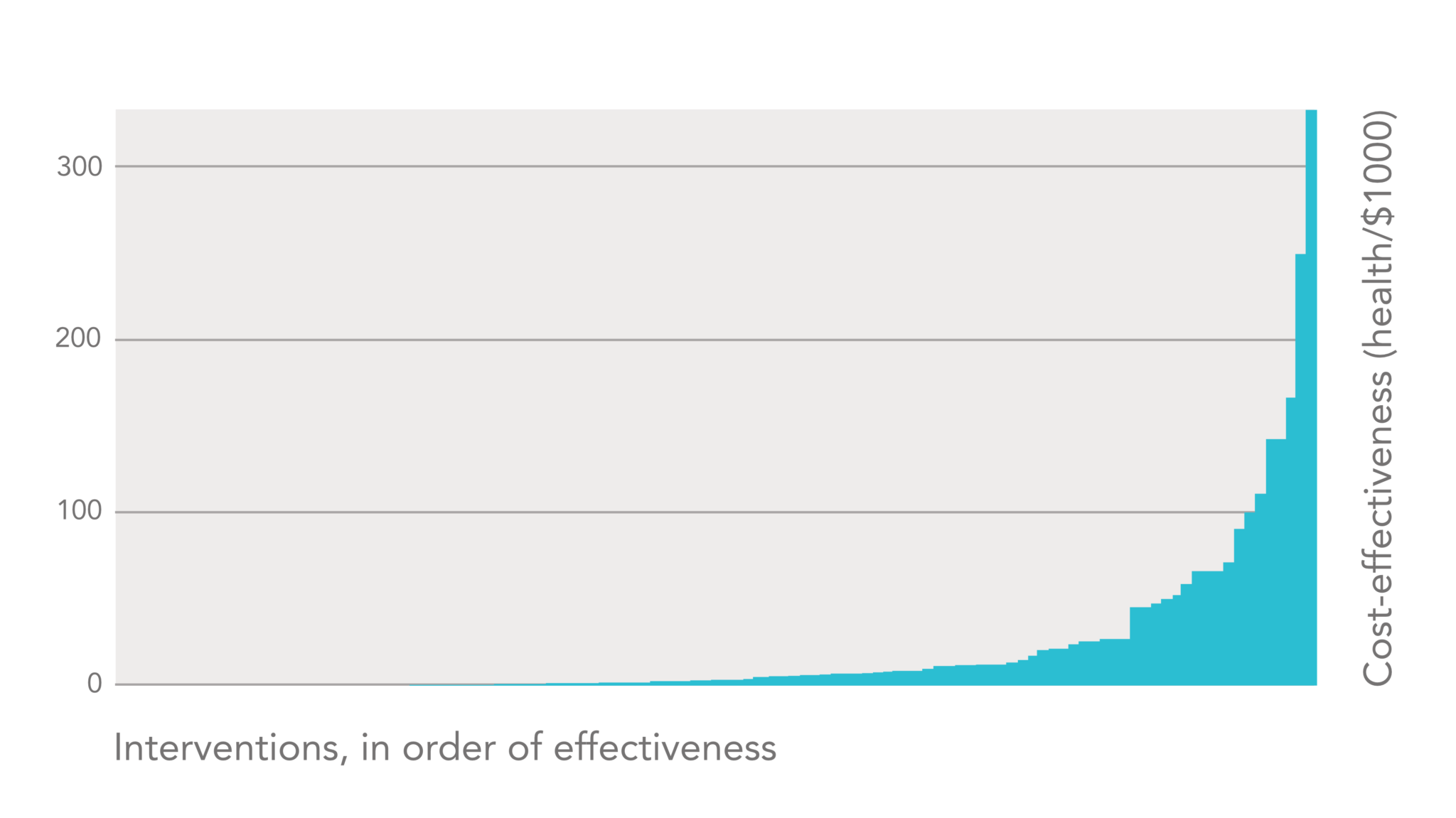
This is an incredible finding, because it suggests that one person working on the most effective interventions within global health could achieve as much as 50 people working on a typical intervention.
We’ve since seem similar patterns among:
- Large US social programmes
- Elsewhere in global health, as well as in health in rich countries
- Education both in the developing and developed world
- Policies to reduce CO2 emissions
- Different ways to get out the vote
We’ve basically found this pattern wherever data is available.
- Other global health datasets, such as WHO-CHOICE and the DCP3
- Public health in rich countries
- Education interventions in developing countries
- Ways to alter meat consumption to reduce animal suffering
- Policies to reduce CO2 emissions
We’ve done a more comprehensive review of the data in a separate research piece:
There are some reasons to think that this data overstates the true differences in effectiveness between different solutions — especially those that you can actually work on going forward.
One reason is that often the most effective solutions in an area are already being done by someone else.
A more subtle reason is regression to the mean. All the estimates involve a lot of uncertainty and error. Some interventions will ‘get lucky’ and end up with errors that make them look better than they are, and others will be unlucky and look worse.
In fact, even if all the solutions were equally effective, random errors would make some look above average, and others below average.
If we compare the interventions that appear best compared to the average, it’s more likely that they benefited from positive errors. This means that if we investigate them more, they’ll probably turn out worse than they seem.
This is what seems to have happened in practice. For instance, the data that Toby used found that distributing insecticide-treated malaria nets was among the most cost-effective solutions in the dataset. However, the latest estimates from the charity evaluator GiveWell are that it’s about 15 times less cost effective than the original estimate.
So, the original estimates were too optimistic, and overstated the spread from best to typical, but the expected cost effectiveness of malaria nets still appears to be much higher than average — likely still over 10 times better.
In fact, global health experts still believe that the best ways of saving lives in poor countries are around 100 times cheaper than the average.4
On the other hand, the data in these studies could also understate the true differences in effectiveness between solutions. One reason is that the data only covers solutions with easy-to-measure results that can be studied in trials, but the highest-impact ways of doing good in the past most often involved research or advocacy, rather than measurable interventions. This would mean the very best solutions are missing from the datasets.
For instance, a comparatively small number of people worked on the development of oral rehydration therapy, which now saves around 1 million lives per year. This research was likely extremely cost effective, but we couldn’t directly measure its effectiveness before it was done.
Looking forward, we think there’s a good case that medical research aimed at helping the global poor will ultimately be more cost effective than spending on direct treatments, increasing the overall degree of spread.
There’s a lot more to say about how much solutions differ in effectiveness, and we’d like to see more research on it. However, our overall judgement is that it’s often possible to find solutions that bring about 10 times as much progress per year of effort than other commonly supported solutions in an area, and it’s sometimes possible to find solutions that achieve 100 times as much.
We go into this question in a lot more detail in the research article mentioned earlier.
Technical aside: theoretical arguments about how much solutions differ
You might think that it’s surprising that such large differences exist. But there are some theoretical arguments that it’s what we should expect:
There isn’t much reason to expect the world of doing good to be ‘efficient’ in the same way that financial markets are, because there are only very weak feedback loops between having an impact and gaining more resources. The main reward people get from doing good is often praise and a sense of satisfaction, but these don’t track the actions that are most effective. We don’t expect it to be entirely inefficient either — even a small number of effectiveness-minded actors can take the best opportunities — but we shouldn’t be surprised to find large differences.
A relatively simple model can give a large spread. For instance, cost effectiveness is produced by the multiple of two factors; we’d expect it to have a log-normal distribution, which is heavy-tailed.
Heavy-tailed distributions seem the norm in many similar cases, for instance how much different experts produce in a field, which means we shouldn’t be surprised if they come up in the world of doing good.
If we think there’s some chance the distribution is heavy-tailed and some chance it’s normally distributed, then in expectation it will be heavy-tailed, and we should act as if it is.
What do these findings imply?
If you’re trying to tackle a problem, it’s vital to look for the very best solutions in an area, rather than those that are just ‘good.’
This contrasts with the common attitude that what matters is trying to ‘make a difference,’ or that ‘every little bit helps.’ If some solutions achieve 100 times more per year of effort, then it really matters that we try to find those that make the most difference to the problem.
So, when comparing how much different careers let you contribute to pressing problems, one key thing to consider is the effectiveness of solutions that you’re able to support. (The other factor is how much leverage the career gives you, which we discuss here.)
This is especially important later in your career when the focus moves from exploring and investing in your skills to deploying your skills and resources on the most pressing problems.
So, how can we find the most effective solutions in an area?
Hits-based vs data-driven approaches
There are two broad approaches among our readers:
- The data-driven approach: look for data about how much progress per dollar different solutions achieve, ideally randomised trials, and focus on the best ones.
- The hits-based approach: look for rules of thumb that make a solution more likely to be among the very best in an area, while accepting that most of the solutions will be duds.
We generally favour the hits-based approach, especially for individuals rather than large institutions, and for people who are able to stay motivated despite a high chance of failure.
Why? As noted, the best solutions typically can’t be measured with trials, and so will be automatically excluded if you take the data-driven approach. This is a serious problem — if the best solutions are far more effective than typical, it could be better to pick randomly among solutions that might be the very best, rather than to pick something that’s very likely to be better than average but definitely not among the very best. And we can probably do significantly better than picking randomly — as covered below.
Another argument is that many institutions with social missions seem overly risk-averse. For instance, government grant agencies get criticised heavily for funding failures, but the employees at such agencies don’t get much reward when they back winners. This suggests that as individuals who are willing to take risks, you can get an edge by supporting solutions that have a high chance of not working. (You can read more about the arguments for a hits-based approach.)
In practice, when it comes to the problem areas we’re most familiar with, the most promising hits-based solutions seem more cost-effective than the most promising data-driven ones.
We don’t believe the hits-based approach is always best. Sometimes it might not be possible to find a hits-based solution that seems better than the best data-driven ones.
For instance, within their global health and wellbeing team, Open Philanthropy believes that while the best hits-based interventions are indeed more effective than the best data-driven ones, they’re not able to find enough of these to fill up all their grantmaking capacity, so in practice they support both data-driven and hits-based approaches.5
Similarly, we’d encourage readers to start by looking for promising solutions with a hits-based approach, and focus on a data-driven one if you’re not able to.
Furthermore, if we take a community perspective, we wouldn’t want 100% of our readers to take a hits-based approach. Collectively we’ll have more impact if a minority specialise in a data-driven approach. (Learn more about worldview diversification.)
One response to the hits-based approach is that it relies on deeply uncertain judgement calls, instead of objective evidence. That’s true, but we contend that you can’t escape relying on judgement calls. All our actions lead to ripple effects lasting long into the future. By taking a data-driven approach, even if we suppose the data is fully reliable, at best it can capture some of the short-term effects. But you’ll need to rely on judgement calls about the longer-term effects — and if we place some value on future generations, the longer-term effects comprise the majority of all the effects.
Given that judgement calls are unavoidable, the best we can do is to try to make the best judgement calls possible, using the best available techniques.
Learn more about this argument and the idea of ‘cluelessness’.
What does taking a hits-based approach involve in practice?
In short, we need to seek rules of thumb that make a solution more likely to be among the very best in an area (and unlikely to be very negative). This could involve methods like the following:
- Upside/downside analysis: look for solutions with potentially very high upsides (and limited downsides).
- Apply the importance, neglectedness, and tractability framework, but at the level of solutions rather than problems. Here’s an example of this kind of analysis within climate change by Founders Pledge.
- Make rough back-of-the-envelope estimates of progress per year of effort invested. Even when the estimates are very uncertain, they can still help us spot very large differences in effectiveness. You can further improve your estimates by applying best practices in forecasting. See some examples from Open Philanthropy.
- Bottleneck analysis: try to identify the key step that would unlock progress in an area.6 For instance, in new areas, it’s often most important to do direction-setting research and demonstrate progress is possible; then the priority becomes building a movement around the issue and scaling up the best solutions.
- Develop a theory of change, and use it to spot key intervention points.
- Taking a data-driven approach can be one input into a hit-based approach: the data-driven approach can help us rule out some interventions that don’t work at all. If we can, say, remove the bottom half of interventions, that can enable us to roughly double our impact, so it’s a useful heuristic – though it’s important to focus more on upsides.
In practice, this often ends up with a focus on research, movement building, policy change, or social advocacy, and on solutions that are unfairly neglected or seem unconventional, or that might have especially high upsides.
In applying these frameworks, you can either try to do this analysis yourself, or find experts in the area who understand the need to prioritise and can do the analysis on your behalf (or a mix of both).
We’re generalists rather than experts in the areas we recommend, so we mainly try to identify good experts — such as those on our podcast — and synthesise their views about how to tackle the problems we write about in our problem profiles.
If you aim your career at tackling a specific issue, however, then you’ll probably end up knowing more about it than us, and so should put more weight on your own analysis.
Many of the areas we recommend are also small, so not much is known about how best to tackle them. This makes it easier than it seems to become an expert. It also means that your input on which solutions are best is especially valuable — one extra person’s views can add significantly to the collective wisdom on the question.
Following expert views also doesn’t necessarily mean choosing ‘consensus’ picks, because those might fall into the trap of being pretty good but not best. Rather, if a minority of experts strongly supports an intervention (and the others don’t think it’s harmful), that might be enough to make it worth betting on. In brief, we aim to consider both the strength of views and how good the interventions seem, and are willing to bet on something contrarian if the upsides might be high enough.
By looking for the best solutions, you can dramatically increase your chances of having a big impact on your chosen problem.
Now go on to read about some career paths that can set you up to get a lot of leverage on the most effective solutions.
Learn more
Top recommendations
- Our more in-depth review on this topic: How much do solutions to social problems differ in their effectiveness? A collection of all the studies we could find
- Hits-based giving — a blog post by Holden Karnofsky at Open Philanthropy.
- Improving global health in clear and direct ways — podcast with Alexander Berger from Open Philanthropy, where he compares evidence-based ways to reduce global poverty with hits-based ones. He claims that while the best hits-based approaches are sometimes better, they can’t find enough of them to deploy their entire budget, so they also support evidence-based ones.
- Is it fair to say most social programmes don’t work?
- Quiz: Can you guess which social programmes work?
Good judgement: why it matters for doing good and how to improve it
Do social science findings generalise? — a podcast with Eva Vivalt
- Expected value estimates you can take (somewhat) literally
— a blog post by Greg Lewis, which explains one way we might try to correct for regression to the mean. - See our list of problem profiles with thoughts on how to best tackle the issues we recommend.
- Search our podcast interviews by issue to hear experts discussing their views.
Read next
This article is part of our advanced series. See the full series, or keep reading:
Notes and references
- van der Put, Claudia E., et al. “Effects of awareness programs on juvenile delinquency: A three-level meta-analysis.” International Journal of Offender Therapy and Comparative Criminology, vol. 65, no. 1, 1996, pp. 68-91. Archived link
Petrosino, Anthony, et al. “Scared Straight and other juvenile awareness programs for preventing juvenile delinquency: A systematic review.” Campbell Systematic Reviews, vol. 9, no.1, (2013), pp. 1-55. Archived link↩ - The percentage that work or don’t work depends a lot on how you define it, but it’s likely that a majority don’t have statistically significant effects.↩
- According to the 2020 Founders Pledge Climate & Lifestyle Report, just one round trip transatlantic flight contributes 1.6 tonnes of CO2. Figure 2 of the same report shows the comparatively negligible effect of reusing plastic bags.↩
- Caviola, Lucius, et al. “Donors vastly underestimate differences in charities’ effectiveness.” Judgment and Decision Making, vol. 15, no. 4, 2020, pp. 509-516. Link
We selected experts in areas relevant to the estimation of global poverty charity effectiveness, in areas such as health economics, international development and charity measurement and evaluation. The experts were identified through searches in published academic literature on global poverty intervention effectiveness and among professional organizations working in charity evaluation.
We found that their median response was a cost-effectiveness ratio of 100 (see Table 1).↩
- Open Philanthropy is 80,000 Hours’ biggest funder as of 2023.↩
- Some common types of bottleneck:
- Funding: additional financial resources from donations or fundraising.
- Insights: new ideas about how to solve the problem.
- Awareness and support: how many people know and care about the issue, and how influential they are.
- Political capital: the amount of political power that’s available for the issue.
- Coordination: the extent to which existing resources effectively work together.
- Community building: finding other people who want to work on the issue.
- Logistics and operations: the extent to which programmes can be delivered at scale.
- Leadership and management: the extent to which concrete plans can be formed and executed using the resources already available.↩