Improving decision making (especially in important institutions)
Ambrogio Lorenzetti, Allegory of Good Government. Photo by Steven Zucker CC BY-NC-SA 2.0
Summary
Working to help governments and other important institutions improve their decision making in complex, high-stakes decisions — especially relating to global catastrophic risks — could potentially be among the most important problems to work on. But there’s a lot of uncertainty about how tractable this problem is to work on and what the best solutions to implement would be.
Our overall view
Recommended
We think working on this issue may be among the best ways of improving prospects for the long-term future — though we’re not as confident in this area as we are in others, in part because we’re not sure what’s most valuable within it.
Scale
We think this kind of work could have a large positive impact. Improvements could lead to more effective allocation of resources by foundations and governments, faster progress on some of the world’s most pressing problems, reduced risks from emerging technologies, or reduced risks of conflict. And if this work could reduce the likelihood of an existential catastrophe — which doesn’t seem out of the question — it could be one of the best ways to improve the prospects for the long-term future.
Neglectedness
Parts of this issue seem extremely neglected. For the sorts of interventions we’re most excited about, we’d guess there are ~100–1,000 people working on them full-time, depending on how you count. But how neglected this makes the issue overall is unclear. Many, many more researchers and consultancies work on improving decision making broadly (e.g. by helping companies hire better). And many existing actors have vested interests in how institutions make decisions, which may cause them to resist certain reforms.
Solvability
Making progress on improving decision making in high-stakes situations seems moderately tractable. There are techniques that we have some evidence can improve decision making, and past track records suggest more research funding directed to the best researchers in this area could be quite fruitful. However, some of these techniques might soon hit a wall in their usefulness, and it’s unclear how easy it will be to get improved decision-making practices implemented in crucial institutions.
Profile depth
Shallow
This is one of many profiles we've written to help people find the most pressing problems they can solve with their careers. Learn more about how we compare different problems, see how we try to score them numerically, and see how this problem compares to the others we've considered so far.
Table of Contents
What is this issue?
Our ability to solve problems in the world relies heavily on our ability to understand them and make high-quality decisions. We need to be able to identify what problems to work on, to understand what factors contribute towards these problems, to predict which of our actions will have the desired outcomes, and to respond to feedback and change our minds.
Many of the most important problems in the world are incredibly complicated, and require an understanding of complex interrelated systems, the ability to make reasonable predictions about the outcome of different actions, and the ability to balance competing considerations and bring different parties together to solve them. That means there are a lot of opportunities for errors in judgement to slip in.
Moreover, in many areas there can be substantial uncertainty about even whether something would be good or bad — for example, does working on large, cutting-edge models in order to better understand them and align their goals with human values overall increase or decrease risk from AI? Better ways of answering these questions would be extremely valuable.
Informed decision making is hard. Even experts in politics sometimes do worse than simple actuarial predictions, when estimating the probabilities of events up to five years in the future.1 And the organisations best placed to solve the world’s most important problems — such as governments — are also often highly bureaucratic, meaning that decision-makers face many constraints and competing incentives, which are not always aligned with better decision making.
There are also large epistemic challenges — challenges having to do with how to interpret information and reasoning — involved in understanding and tackling world problems. For example, how do you put a probability on an unprecedented event? How do you change your beliefs over time — e.g. about the level of risk in a field like AI — when informed people disagree about the implications of developments in the field? How do you decide between, or balance, the views of different people with different information (or partially overlapping information)? How much trust should we have in different kinds of arguments?
And what decision-making tools, rules, structures, and heuristics can best set up organisations to systematically increase the chances of good outcomes for the long-run future — which we think is extremely important? How can we make sensible decisions, given how hard it is to predict events even 5 or 10 years in the future?2
And are there better processes for implementing those decisions, where the same conclusions can lead to better results?
We think that improving the internal reasoning and decision making competence of key institutions — some government agencies, powerful companies, and actors working with risky technologies — is particularly crucial. If we’re right that the risks we face as a society are substantial, and these institutions can have an outsized role in managing them, the large scale of their impact makes even small improvements high-leverage.
So the challenge of improving decision making spans multiple problem areas. Better epistemics and decision making seems important in reducing the chance of great power conflict, in understanding risks from AI and figuring out what to do about them, in designing policy to help prevent catastrophic pandemics, in coordinating to fight climate change, and basically every other difficult, high-stakes area where there are multiple actors with incomplete information trying to coordinate to make progress — that is, most of the issues we think are most pressing.
What parts of the problem seem most promising to work on?
‘Improving decision making’ is a broad umbrella term. Unfortunately, people we’ve spoken to disagree a lot on what more specifically is best to focus on. Because of this lack of clarity and consensus, we have some scepticism that this issue as we’ve defined it is as pressing as better-defined problems like AI safety and nuclear risk.
However, some of the ideas in this space are exciting enough that we think they hold significant promise, even if we’re not as confident in recommending that people work on them as we are for other interventions.
Here are some (partially overlapping) areas that could be particularly worth working on:
Forecasting
A new science of forecasting is developing around improving people’s ability to make better all-things-considered predictions about future events by developing tools and techniques as well as ways of combining them into aggregate predictions. This field tries to answer questions like, for example, what’s the chance that the war in Ukraine ends in 2023? (It’s still going at the time of this writing), or what’s the chance that a company will be able to build an AI system that can act as a commercially viable ‘AI executive assistant’ by 2030?
Definitions are slippery here, and a lot of things are called ‘forecasting.’ For example, businesses forecast supply and demand, spending, and other factors important for their bottom lines all the time. What seems special about some kinds of forecasting is a focus on finding the best ways to get informed, all-things-considered predictions for events that are really important but complex, unprecedented, or otherwise difficult to forecast with established methods.
One approach in this category is Dr Phil Tetlock’s search for ‘superforecasters’ — people who have a demonstrated track record of being unusually good at predicting events. Tetlock and his collaborators have looked at what these superforecasters are actually doing in practice to make such good predictions, and experimented with ways of improving on their performance. Two of their findings: the best forecasters tended to take in many different kinds of information and combine them into an overall guess, rather than focusing on e.g. a few studies or extrapolating one trend; and an aggregation of superforecasters’ predictions tended to be even more accurate than the best single forecasters on their own.
Developing and implementing better forecasting of important events and milestones in technological progress would be extremely helpful for making more informed high stakes decisions, and this new science is starting to gain traction. For example, the US’s Intelligence Advanced Research Projects Activity (IARPA) took an interest in the field in 2011 when it ran a forecasting tournament. The winning team was Tetlock’s, the Good Judgement Project, which has turned into a commercial forecasting outfit, Good Judgement Inc.
Here’s a different sort of example of using forecasting from our own experience: one of the biggest issues we aim to help people work on is reducing existential threats from transformative AI. But most work in this area is likely to be more useful before AI systems reach transformative levels of power and intelligence. That makes when this transition happens (which may be gradual) an important strategic consideration. If we thought transformative AI was just around the corner, it’d make more sense for us to try to work with mid-career professionals to pivot to working on the issue, since they already have lots of experience. But if we have a few decades, there’s time to help a new generation of interested people to enter the field and skill up.
Given this situation, we keep an eye on forecasts from researchers in AI safety and ML; for example, Ajeya Cotra’s forecasts based on empirical scaling laws and biological anchors (like the computational requirements of the human brain), expert surveys, and forecasts by a ‘superforecasting’ group called Samotsvety. Each of these forecasts uses a different methodology and is highly uncertain, and it’s unclear which is best; but they’re the best resource we have for this decision. Together and so far, forecasts suggest there’s a decent probability that there is enough time for us to help younger people enter careers in the field and start contributing. But more and better forecasting in this area, as well as more guidance on best practices for how to combine different forecasts, would be highly valuable and decision-relevant.
Policy is another area where forecasting seems important. The forecasted effects of different proposed policies are the biggest ingredient determining their value — but these predictions are often very flawed. Of course, this isn’t the only thing that stands in the way of better policymaking — for example, sometimes good policies hit political roadblocks, or just need more research. But predicting the complex effects of different policies is one of the fundamental challenges of governance and civil society alike.
So, improving our ability to forecast important and complex events seems robustly useful. However, the most progress has so far been made on relatively well-defined predictions in the near term future. It’s as-yet unproven that we can reliably make substantially better-than-chance predictions about more complex or amorphous issues or events greater than a few years in the future, like, for example, “when, if ever, will AI transform the economy?”
And we also don’t know much about the extent to which better forecasting methods might improve decision making in practice, especially in large, institutional settings. What are the best and most realistic ways the most important agencies, nonprofits, and companies can systematically use forecasting to improve their decision making? What proposals will be most appealing to leadership, and easiest to implement?
It also seems possible this new science will hit a wall. Maybe we’ve learned most of what we’re going to learn, or maybe getting techniques adopted is too intractable to be worth trying. We just don’t know yet.
So: we’re not sure to what extent work on improving forecasting will be able to help solve big global problems — but the area is small and new enough that it seems possible to make a substantial difference on the margin, and the development of this field could turn out to be very valuable.
Prediction aggregators and prediction markets
Prediction markets use market mechanisms to aggregate many people’s views on whether some or another event will come about. For example, the prediction markets Manifold Markets and Polymarket let users bet on or forecast on questions like “who will win the US presidential election in 2024?” or even “Will we see a nuclear detonation by mid-2023?”. Users who correctly predict events can earn money, and can earn bigger returns in proportion to how unexpected the outcome they predicted was.
The idea is that prediction markets provide a strong incentive for individuals to make accurate predictions about the future. In the aggregate, the trends in these predictions should provide useful information about the likelihood of the future outcomes in question — assuming the market is well designed and functioning properly.
There are also other prediction aggregators, like Metaculus, that use reputation as an incentive rather than money (though Metaculus also hosts forecasting tournaments with cash prizes), and which specialise in aggregating and weighting predictions by track records to improve collective forecasts.
Prediction markets in theory are very accurate when there is a high volume of users, in the same way the stock market is very good at valuing shares in companies. In the ideal scenario, they might be able to provide an authoritative, shared picture of the world’s aggregate knowledge on many topics. Imagine never disagreeing on what “experts think” at the Thanksgiving table again — then imagine those disagreements evaporating in the White House situation room.
But prediction markets or other kinds of aggregators don’t exist yet on a large scale for many topics, so the promise is still largely theoretical. They also wouldn’t be a magic bullet: like stock markets, we might see them move but not know why; and they wouldn’t tell us much about topics where people don’t have much reason to think one thing or another. Likewise, they add another set of complex incentives to political decision making — many prediction markets can also incentivise the things they predict. Moreover, even if large and accurate prediction markets were created, would the most important institutional actors take them into account?
These are big challenges, but the theoretical arguments in favour of prediction markets and other aggregators are compelling, and we’d be excited to see markets gain enough traction to have a full test of their potential benefits.
Decision-making science
Another category here is developing tools and techniques for making better decisions — for example ‘structured analytic techniques’ (SATs) like the Delphi method, which helps build consensus in groups by using multiple iterations of questions to collect data from different group members, and the creation of crowdsourcing techniques like SWARM (‘Smartly-assembled Wiki-style Argument Marshalling’). (You can browse uses of the Delphi method on the website of the RAND corporation, which first developed the method in the 1950s.)
These tools might help people avoid cognitive failings like confirmation bias, help aggregate views or reach consensus, or help stop ‘information cascades’.
Many of these techniques are already in use. But it seems likely that better tools could be developed, and it’d be very surprising if there weren’t more institutional settings where the right techniques could be introduced.
Improving corporate governance at key private institutions
Corporate governance might be especially important as we develop transformative and potentially dangerous technologies in the fields of AI and bioengineering.
Decision making responds to incentives, which can often be affected by a company’s structure and governance. For example, for-profit companies are ultimately expected to maximise shareholder profit — even if that has large negative effects on others (‘externalities’) — whereas nonprofits are not.
As an example, the AI lab OpenAI set up its governance structure with the explicit goal that profits not take precedence over the mission codified in its charter (that general AI systems benefit all of humanity), by putting its commercial entity under the control of its nonprofit’s board of directors.
We’re not sure this is doing enough to reduce the chance of negative externalities from OpenAI’s operations, and take seriously concerns raised by others about the company’s actions potentially leading to arms race dynamics, but it seems better than a traditional commercial setup for shaping corporate incentives in a good direction, and we’d be excited to see more innovations as well as interest from companies in this space. (Though we’re also not sure improving governance mechanisms is more promising than advocacy and education about potential risks — both seem helpful.)
Improving how governments work
Governments control vast resources and are sometimes the only actors that can work on issues of global scale. But their decisions seem substantially shaped by the structures and processes that make them up. If we could improve these processes, especially in high-stakes or fast-moving situations, that might be very valuable.
For example, during the COVID-19 pandemic, reliable rapid tests were not available for a very long time in the US compared to other similar countries, arguably slowing the US’s ability to manage the pandemic. Could better institutional decision-making procedures — especially better procedures for emergency circumstances — have improved the situation?
Addressing this issue would require a detailed knowledge of how complex, bureaucratic institutions like the US government and its regulatory frameworks work. Where exactly was the bottleneck in the case of COVID rapid tests? What are the most important bottlenecks to address before the next pandemic?
A second area here is voting reform. We often elect our leaders with ‘first-past-the-post’-style voting, but many people argue that this leads to perverse outcomes. Different voting methods could lead to more functional and representative governments in general, which could be useful for a range of issues. See our podcast with Aaron Hamlin to learn more.
There is also the importance of voting security to prevent contested elections, discussed in our interview with Bruce Schneier.
Finally there are interventions aimed at getting governance mechanisms to systematically account for the interests of future generations. Right now future people have no commercial or formal political power, so we should expect their interests to be underserved. Some efforts to combat this phenomenon include the Future Generations Commissioner for Wales and the Wellbeing of Future Generations bill in the UK.
Ultimately, we’re unsure what the best levers to push on are here. It seems clear that there are problems with society’s ability to reason about large and complex problems and act appropriately — especially when decisions have to be made quickly, when there are competing motives, when cooperation is needed, and when the issues primarily affect the future. And we’re intrigued by some of the progress we’ve seen.
But some of the best interventions may also be specific to certain problem areas — e.g. working on better forecasting of AI development.
And tractability remains a concern even when better methods are developed. Though there are various examples of institutions adopting techniques to improve decision-making quality, it seems difficult to get others to adopt best practices, especially if the benefits are hard to demonstrate, you don’t understand the organisations well enough, or if they don’t have adequate motivation to adopt better techniques.
What are the major arguments against improving decision making being a pressing issue?
It’s unclear what the best interventions are — and some are not very neglected.
As we covered above, we’re not sure about what’s best in this area.
One reason for doubt about some of the kinds of work that would fall into this bucket is that they seem like they should be taken care of by market mechanisms.
A heuristic we often use for prioritising global problems is: will our economic system incentivise people to solve this issue on its own? If so, it’s less obvious that people should work on it in order to try to improve the world. This is one way of estimating the future neglectedness of an issue. If there’s money to be made by solving a problem, and it’s possible to solve it, it stands a good chance of getting solved.
So one might ask: shouldn’t many businesses have an interest in better decision making, including about important and complex events in the medium and long-run future? Predicting future world developments, aggregating expert views, and generally being able to better make high stakes decisions seems incredibly useful in general, not just for altruistic purposes.
This is a good question, but it applies more to some interventions than others. For example, working to shape the incentives of governments so they better take into account the interests of future generations seems very unlikely to be addressed by the market. Ditto better corporate governance.
Better forecasting methods and prediction markets seem more commercially valuable. Indeed, the fact that they haven’t been developed more under the competitive pressure of the market could be taken as evidence against their effectiveness. Prediction markets, in particular, were first theorised 20 years ago — maybe if they were going to be useful they’d be mainstream already? Though this may be in part because they’re often considered a form of illegal gambling in the US, and our guess is they’re still valuable and are being underrated.
Decision science tools are probably the least neglected of the sub-areas discussed above — and, in practice, many industries do use these methods, and think tanks and consultancies develop and train people in them.
All that said, the overall problem here still seems unsolved, and even methods that seem like they should receive commercial backing haven’t yet caught on. Moreover, our ability to make decisions seems most inadequate in the most high-stakes situations, which often involve thinking about unknown unknowns, small probabilities of catastrophic outcomes, and deep complexity — suggesting more innovation is needed. Plus, though use of different decision-making techniques is scattered throughout society, we’re a long way from all the most important institutions using the best tools available to them.
So though there is broad interest in improving decision making, it’d be surprising to us if there weren’t a lot of room for improvement on the status quo. So ultimately we’re excited to see more work here, despite these doubts.
You might want to just work on a pressing issue more directly.
Suppose you think that climate change is the most important problem in the world today. You might believe that a huge part of why we’re failing to tackle climate change effectively is that people have a bias towards working on concrete, near-term problems over those that are more likely to affect future generations — and that this is a systematic problem with our decision making as a society.
And so you might consider doing research on how to overcome this bias, with the hope that you could make important institutions more likely to tackle climate change.
However, if you think the threat of climate change is especially pressing compared to other problems, this might not be the best way for you to make a difference. Even if you discover a useful technique to reduce the bias to work on immediate problems, it might be very hard to implement it in a way that directly reduces the impacts of climate change.
In this case, it’s likely better to focus your efforts on climate change more directly — for example by working for a think tank doing research into the most effective ways to cut carbon emissions, or developing green tech. In general, more direct interventions seem more likely to move the needle on particular problems because they are more focused on the most pressing bottlenecks in that area.
That said, if you can’t implement solutions to a problem without improving the reasoning and decision-making processes involved, it may be a necessary step.
The chief advantage of broad interventions like improving decision making is that they can be applied to a wide range of problems. The corresponding disadvantage is that it might be harder to target your efforts towards a specific problem. So if you think one specific problem is significantly more urgent than others, and you have an opportunity to work on that problem more directly, then it is likely more effective to do the direct work.
It’s worth noting that in some cases this is not a particularly meaningful distinction. Developing good corporate governance mechanisms that allows companies developing high-stakes technology to better cooperate with each other is a way of improving decision making. But it may also be one of the best ways to directly reduce catastrophic risks from AI.
It’s often difficult to get change in practice
Perhaps the main concern with this area is that it’s not clear how easy it is to actually get better decision-making strategies implemented in practice — especially in bureaucratic organisations, and where incentives are not geared towards accuracy.
It’s often hard to get groups to implement practices that are costly to them in the short term — requiring effort, resources, and sometimes challenges current stakeholders — while only promising abstract or long-term benefits. There can also be other constraints — as mentioned above, running prediction markets with real money is usually considered illegal in the US.
However, implementation problems may be surmountable if you can show decision-makers that the techniques will help them achieve the objectives they care about.
If you work at or are otherwise able to influence an institution’s setup, you might also be able to help shift decision-making practices by setting up institutional incentives that favour deliberation and truth-seeking.
But recognising the difficulties of getting change in practice also means it seems especially valuable for people thinking about this issue to develop an in-depth understanding of how important groups and institutions operate, and the kinds of incentives and barriers they face. It seems plausible that overcoming bureaucratic barriers to better decision making may be even more important than developing better techniques.
There might be better ways to improve our ability to solve the world’s problems
One of the main arguments for working in this area is that if you can improve the decision-making abilities of people working on important problems, then this increases the effectiveness of everything they do to solve those problems.
But you might think there are better ways to increase the speed or effectiveness of work on the world’s most important problems.
For example, perhaps the biggest bottleneck on solving the world’s problems isn’t poor decision making, but simply lack of information: people may not be working on the world’s biggest problems because they lack crucial information about what those problems are. In this case the more important area might be global priorities research — research to identify the issues where people can make the biggest positive difference.
Also, a lot of work on building effective altruism might fall in this category: giving people better information about the effectiveness of different causes, interventions, and careers, as well as spreading positive values like caring about future generations.
What can you do in this area?
We can think of work in this area as falling into several broad categories:
- More rigorously testing existing techniques that seem promising.
- Doing more fundamental research to identify new techniques.
- Fostering adoption of the best proven techniques in high-impact areas.
- Directing more funding towards the best ways of doing all of the above.
All of these strategies seem promising and seem to have room to make immediate progress (we already know enough to start trying to implement better techniques, but stronger evidence will make adoption easier, for example). This means that which area to focus on will depend quite a lot on your personal fit and the particular opportunities available to you — we discuss each in more detail below.
1. More rigorously testing existing techniques that seem promising
We went through some different areas where you could have very different sorts of interventions above. We think more rigorously testing tentative findings in all those areas — especially ones that are the least developed — could be quite valuable.
Here we’re just going to talk about a few examples of techniques on the more formal end of the spectrum, because it’s what we know more about:
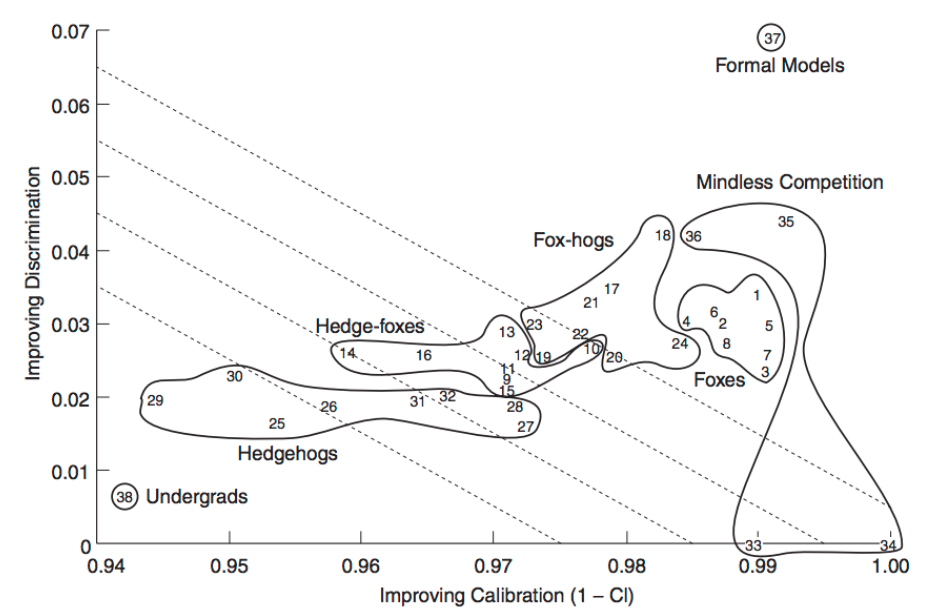
The idea here would be to take techniques that seem promising, but haven’t been rigorously tested yet, and try to get stronger evidence of where and whether they are effective. Some techniques or areas of research that fall into this category:
- Calibration training — one way of improving probability judgements — has a reasonable amount of evidence suggesting it is effective. However, most calibration training focuses on trivia questions — testing whether this training actually improves judgement in real-world scenarios could be promising, and could help to get these techniques applied more widely.
- ‘Pastcasting’ is a method of achieving more realistic forecasting practice by using real forecasting questions – but ones that have been resolved already.
- Structured Analytic Techniques (SATs) — e.g. checking key assumptions and challenging consensus views. These seem to be grounded in an understanding of the psychological literature, but few have been tested rigorously (i.e. with a control group and looking at the impact on accuracy). It could be useful to select some of these techniques that look most promising, and try to test which are actually effective at improving real-world judgements.3 It might be particularly interesting and useful to try to directly pitch some of these techniques against each other and compare their levels of success.
- Methods of aggregating expert judgements, including Roger Cooke‘s classical model for structured expert judgement (which scores different judgements according to their accuracy and informativeness and then uses these scores to combine them),4 prediction markets, or the aforementioned Delphi method.
- Better expert surveying in important areas like AI and biorisk — for example, on the model of the IGM Economic Experts Panel.
A few academic researchers and other groups working in this area:
- Professor Philip Tetlock at the University of Pennsylvania, who we mentioned previously and who has pioneered research in improving forecasting.
- The Forecasting Research institute designs and tests forecasting techniques with a focus on getting them implemented by policymakers and nonprofits.
- Professor Robin Hanson, an economist at George Mason University who has researched and advocated for the use of prediction markets.
- Professor George Wright at Strathclyde University in Glasgow, a psychologist who does research into applying the Delphi method and scenario thinking to improve judgements.
- Stephen Coulthart at the University of Albany has done some research looking at the effectiveness of different SATs in intelligence analysis.5
- There are some groups focused on more applied decision-making research, which may have money to run large field experiments, particularly in business schools, including: the Behavioural Insights Group at the Harvard Kennedy School; the Operations, Information and Decisions Group at the Wharton School, University of Pennsylvania; the Decision Sciences Group at Duke’s Fuqua School of Business; the Center for Decision Research at The University of Chicago Booth School of Business; and the Behavioural Science Group at Warwick Business School.
There may also be some non-academic organisations with funding for, and interest in running, more rigorous tests of known decision-making techniques:
- Intelligence Advanced Research Projects Activity (IARPA) is probably the biggest funder of research in this area right now, especially with a focus on improving high-level decisions.
- Open Philanthropy, which also funds 80,000 Hours, sometimes makes grants aimed at testing and developing better decision-making techniques — especially ones aimed at helping people make better decisions about the future.
- Consultancies with a behavioural science focus, such as the Behavioural Insights Team, may also have funding and interest in doing this kind of research. These organisations generally focus on improving lots of small decisions, rather than on improving the quality of a few very important decisions, but they may do some work on the latter.
2. Doing more fundamental research to identify new techniques
You could also try to do more fundamental research: developing new approaches to improved epistemics and decision making, and then testing them. This is more pressing if you don’t think existing interventions like those listed above are very good.
One example of an open question in this area is: how do we judge ‘good reasoning’ when we don’t have objective answers to a question? That is, when we can’t just judge answers or contributions based on whether they lead to accurate predictions or answers we know to be true?6 Two examples of current research programmes related to this question are IARPA’s Crowdsourcing Evidence, Argumentaion, Thinking and Evaluation (CREATE) programme and Philip Tetlock’s Making Conversations Smarter, Faster (MCSF) project.
The academics and institutions listed above might also be promising places to work or apply for funding if you’re interested in developing new decision-making techniques.
3. Fostering adoption of the best proven techniques in high-impact institutions
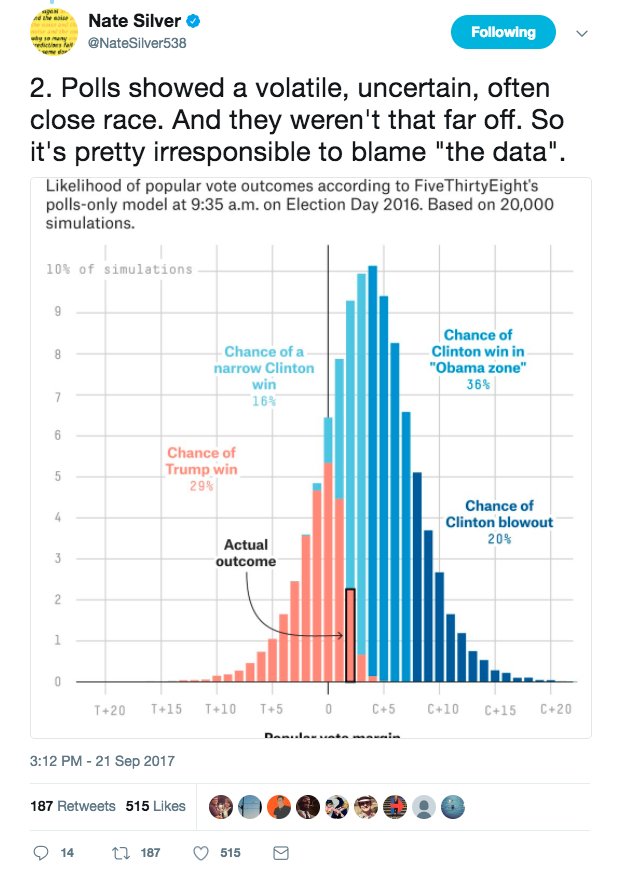
Alternatively, you could focus more on implementing those techniques you currently think are most likely to improve collective decision making (such as the research on forecasting by Tetlock, prediction markets, or SATs).7 If you think one specific problem is particularly important, you might prefer to focus on the implementation of techniques (rather than developing new ones), as this is easier to target towards specific areas.
As mentioned above, a large part of ‘fostering adoption’ might first require better understanding the practical constraints and incentives of different groups working on important problems, in order to understand what changes are likely to be feasible. For this reason, working in any of the organisations or groups listed below — with the aim of better understanding the barriers they face and building connections — might be valuable, even if you don’t expect to be in a position to change decision-making practices immediately.
These efforts might be particularly impactful if focused on organisations that control a lot of resources, or organisations working on important problems. Here are some examples of specific places where it might be good to work if you want to do this:
- Any major government, perhaps especially in the following areas: (1) intelligence/national security and foreign policy; (2) parts of government working on technology policy (the Government Office for Science in the UK, for example, or the Office of Science and Technology Policy in the US); (3) development policy (DFID in the UK, USAID in the US); or (4) defence (the Department of Defense in the US, or the Ministry of Defence in the UK).
- Organisations that direct large amounts of money towards solving important world problems — such as foundations or grants agencies like the Gates Foundation or Open Philanthropy. See also our profile on working as a foundation grantmaker.
- Some international organisations like the UN
- Private companies involved in creating transformative technologies, or the agencies that regulate them
You could also try to test and implement improved decision-making techniques in a range of organisations as a consultant. Some specific organisations where you might be able to do this, or at least build up relevant experience, include:
- Good Judgment, which runs training for individuals and organisations to apply findings from forecasting science to improve predictions.
- Working at a specialist ‘behavioural science’ consultancy, such as the Behavioural Insights Team or Ideas42. Some successful academics in this field have also set up smaller consultancies — such as Hubbard Decision Research (which has worked extensively on calibration training).8
- HyperMind, an organisation focused on wider adoption of prediction markets.
- Going into more general consultancy, with the aim of trying to specialise in helping organisations with better decision making — see our profile on management consulting for more details.
Another approach would be to try to get a job at an organisation you think is doing really important work, and eventually aim to improve their decision making. Hear one story of someone improving a company’s decisionmaking from the inside in our podcast with Danny Hernandez.
Finally, you could also try to advocate for the adoption of better practices across government and highly important organisations like AI or bio labs, or for improved decision making more generally — if you think you can get a good platform for doing so — working as a journalist, speaker, or perhaps an academic in this area.
Julia Galef is an example of someone who has followed this kind of path. Julia worked as a freelance journalist before cofounding the Center for Applied Rationality. She co-hosts the podcast Rationally Speaking, and has a YouTube channel with hundreds of thousands of followers. She’s also written a book we liked, the Scout Mindset, aimed at helping readers maintain their curiosity and avoid defensively entrenching their beliefs. You can learn more about Julia’s career path by checking out our interview with her.
4. Directing more funding towards research in this area
Another approach would be to move a step backwards in the chain and try to direct more funding towards work in all of the aforementioned areas: developing, testing, and implementing better decision-making strategies.
The main place we know of that seems particularly interested in directing more funding towards improving decision-making research is IARPA in the US.9 Becoming a programme manager at IARPA — if you’re a good fit and have ideas about
areas of research that could do with more funding — is therefore a very promising opportunity.
There’s also some chance Open Philanthropy will invest more time or funds in exploring this area (they have previously funded some of Tetlock’s work on forecasting).
Otherwise you could try to work at any other large foundation with an interest in funding scientific research where there might be room to direct funds towards this area.
Special thanks to Jess Whittlestone who wrote the original version of this problem profile, and from whose work much of the above text is adapted.
Learn more
Top recommendations
- Listen to our podcast episodes on forecasting with Philip Tetlock: Prof Tetlock on predicting catastrophes, why keep your politics secret, and when experts know more than you and Accurately predicting the future is central to absolutely everything. Professor Tetlock has spent 40 years studying how to do it better.
- Learn about Our World in Data’s strategy for making important information about the biggest issues out there available to decision-makers everywhere.
- Read Lizka Vaintrob’s blog post, Disentangling “Improving Institutional Decision-Making.”
- We also recommend our podcast with Mushtaq Khan on using institutional economics to predict effective government reforms and our episode with Danny Hernandez on forecasting AI progress.
- See also this video interview with 80,000 Hours advisor Alex Lawsen on forecasting and AI progress.
- For discussion of some of the other issues in this space, check out our podcast episodes on voting reform with Aaron Hamlin, Taiwan’s experiments with democracy with Audrey Tang, and misaligned incentives in international development with Karen Levy.
See a talk by Tyler John on ways governments can take into account the interests of future generations.
- If you’re interested in doing research in this area, our career profile on valuable academic research gives more detail on that general career path.
- To learn more about existing research in this area, consider reading: (1) Philip Telock’s Superforecasting, (2) Robin Hanson’s work promoting prediction markets, (3) some of the other research emerging from IARPA’s relevant grants, in particular: ACE, HFC, FUSE, and SHARP.
- See also the work of Andy Matuschak, Michael Nielsen, and Bret Victor on “tools for thought”, new mediums for science and engineering, and “programmable attention”. Also this blog post about their work, by a former Chief Advisor to a UK Prime Minister.
Get free, one-on-one career advice
We’ve helped hundreds of people compare their options, get introductions, and get jobs important for the long-run future. Find out if our coaching can help you:
Notes and references
- “In political forecasting, Tetlock (2005) asked professionals to estimate the probabilities of events up to 5 years into the future – from the standpoint of 1988. Would there be a nonviolent end to apartheid in South Africa? Would Gorbachev be ousted in a coup? Would the United States go to war in the Persian Gulf? Experts were frequently hard-pressed to beat simple actuarial models or even chance baselines (see also Green and Armstrong, 2007).” Mellers et. al. (2015). The Psychology of Intelligence Analysis: Drivers of Prediction Accuracy in World Politics. The Journal of Experimental Psychology: Applied. 21, 1, pp. 1-14↩
- See this blog post for an attempt to assess performance differences in predictions over longer and shorter time horizons. Unfortunately there isn’t much to go on, because we have very little public data on predictions more than one year in the future.↩
- The biggest programme we’ve heard of testing the effectiveness of different SATs was the CREATE program, funded by IARPA.↩
- Source↩
- Source↩
- Many of the most important kinds of decisions policymakers have to make aren’t questions with clear, objective answers, and we don’t currently have very good ways to judge the quality of reasoning in these cases. Effective solutions to this seem like they could be very high impact, but we don’t currently know whether this is possible or what they would look like.↩
- Note there’s some overlap here with more rigorously testing existing techniques since part of what’s required to foster adoption will be providing people with evidence that they work! So going to work at a more ‘applied’ organisation with an interest in rigorous evaluation might provide an opportunity to work on both getting better evidence for existing techniques, and getting them implemented.↩
- It’s worth noting, though, that many of these consultancies work mostly with corporate clients, and so this might not be the best opportunity for immediate impact — but it might be a good way to test out improved decision-making strategies in different environments, from which we could learn about how to apply these techniques in more important areas.↩
- IARPA was the main funder behind a lot of the research on forecasting, for example, and have a number of past and open projects focused on this area, including ACE, FUSE, SHARP, and OSI.↩