Expert in AI hardware
Credit: Fritzchens Fritz CC0
In 1965, Gordon Moore observed that the number of transistors you can fit onto a chip seemed to double every year. He boldly predicted, “Integrated circuits will lead to such wonders as home computers[,] automatic controls for automobiles, and personal portable communications equipment.”1
Moore later revised his estimate to every two years, but the doubling trend held, eventually becoming known as Moore’s Law.
This technological progress in computer hardware led to consistent doublings of performance, memory capacity, and energy efficiency. This was achieved only through astonishing increases in the complexity of design and production. While Moore was looking at chips with fewer than a hundred transistors, modern chips have transistor counts in the tens of billions and can only be fabricated by some of the most complex machinery humans have invented.2
Besides personal computers and mobile phones, these enormous gains in computational resources — “compute” — have also been key to today’s rapid advances in artificial intelligence. Training a frontier model like OpenAI’s GPT-4 requires thousands of specialised AI chips with tens of billions of transistors, which can cost tens of thousands of dollars each.3
As we have outlined in our AI risk problem profile, we think dangers from advanced AI are among the most pressing problems in the world. As they progress this century, AI systems — created with and running on AI hardware — may develop advanced capabilities and features that carry profound risks for humanity’s future.
Navigating those risks will require crucial work in forecasting AI progress, researching and implementing governance mechanisms, and assisting policy makers, among other things. Expertise in AI hardware can be of use in all these activities.
We are very enthusiastic about altruistically motivated people who already have AI hardware expertise moving into the AI governance and policy space in the short term. And we’re also enthusiastic about people with the background skills and strong personal fit to succeed in this field gaining AI hardware expertise and experience that could be useful later on.
Using AI hardware expertise to reduce catastrophic risks is a relatively new field, and there is a lot of work needed right now to develop it.
It’s hard to predict how the field will evolve, but we’d guess that there will continue to be useful ways to contribute for years to come. At some point, there may be less need for conceiving governance regimes and more work needed working out implementation details of specific policies. So we’re also pretty comfortable recommending people start now on gaining hardware-related skills and experience that could be useful later on. Hardware skills and experience are highly valuable in general, so this path is likely to have good exit options anyway.
You can also read our career review of AI governance and coordination, which discusses how valuable this kind of expertise can be for policy.
Table of Contents
In a nutshell: Reducing risks from AI is one of the most pressing problems in the world, and we expect people with expertise in AI hardware and related topics will be in particularly high demand in policy and research in this area. For the right person, gaining and applying AI hardware skills to risk-reducing AI governance work could be their most impactful option.
But becoming an expert in this field is not easy and will not be a good fit for most people, and it may be challenging to chart a clear path through the complex and evolving world of AI governance agendas.
Pros
- Opportunity to make a significant contribution to the growing field of AI governance
- Intellectually challenging work that offers strong career capital for a range of paths
- Working in a cutting-edge and fast-moving area
Cons
- You need strong quantitative and technical skills
- There’s a lot of uncertainty about what needs to be done in this space
- There’s a real possibility of causing harm in this field
- Some — but not all — of the relevant roles may involve stressful work and long hours
Key takeaways on fit
For anyone with expertise in AI hardware, using these skills to contribute to risk-reducing governance approaches should be a top contender for your career. If you don’t yet have this experience, it might be worth developing these skills if you’re particularly excited about studying computer science and engineering, electrical engineering, or other relevant fields. These fields require strong maths and science skills.
We suggest anyone interested in this path should also familiarise themselves with AI governance and coordination.
Recommended
If you are well suited to this career, it may be the best way for you to have a social impact.
Review status
Based on a medium-depth investigation
Why might becoming an expert in AI hardware be high impact?
The basic argument for why being an expert in AI hardware could be impactful is:
- Increasingly advanced AI seems likely to be very consequential this century and may carry existential risks.
- There are various ways that expertise in AI hardware can help with (a) forecasting AI progress and (b) ensuring AI is developed responsibly.
The main reason why expertise in AI hardware can help reduce risk is that, alongside data and ideas, compute is a key input into overall AI progress.4
Researchers have identified scaling laws showing that, as you train AI systems using more compute and data, those systems predictably improve on many performance metrics. As a result, you now need thousands of expensive AI chips running for months to train a frontier AI model, amounting to tens of millions of dollars in compute costs alone.5
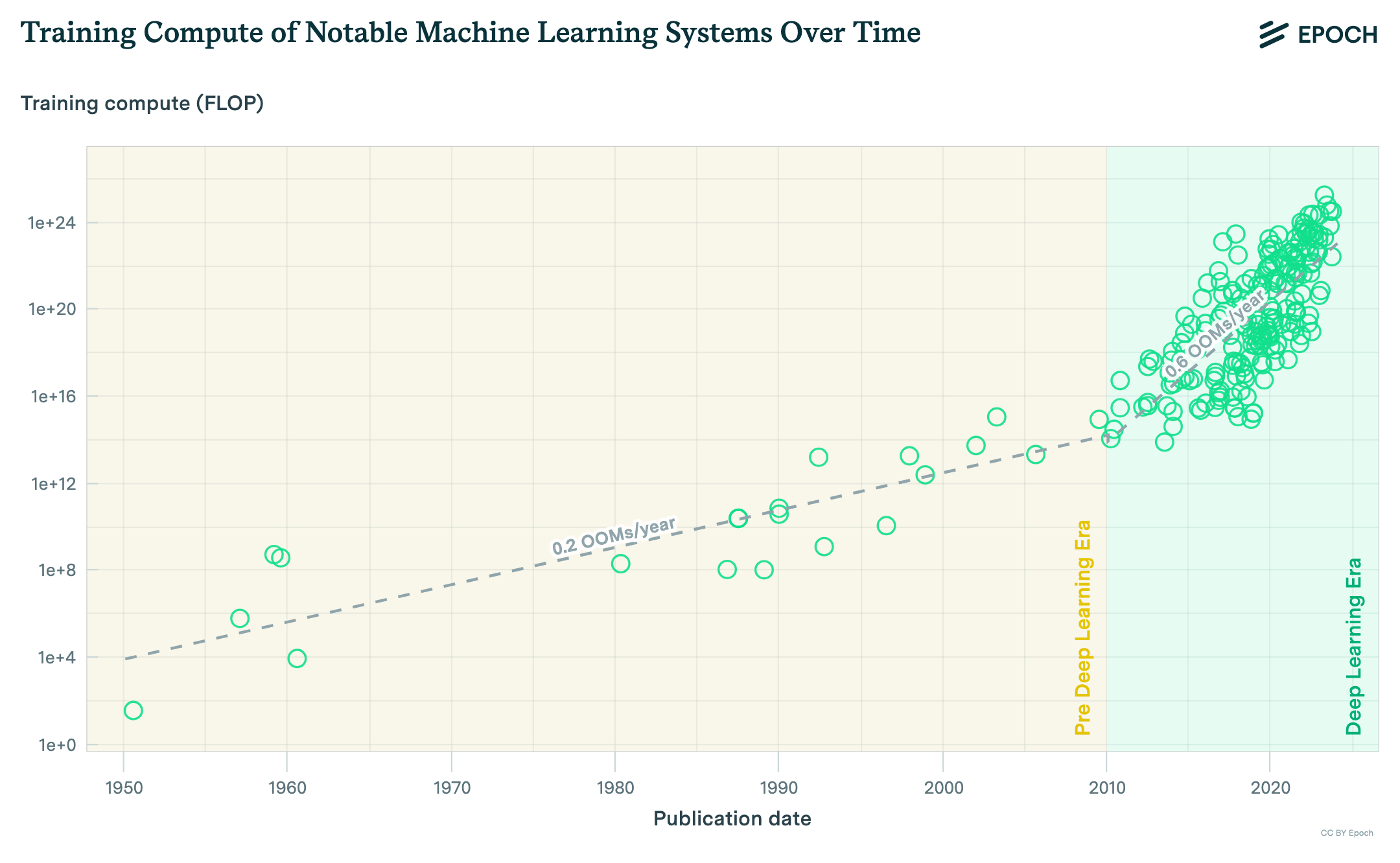
Credit: Epoch AI (2023)
AI chips are specialised to perform the specific calculations needed for training and running AI models. In practice, you cannot train frontier models with general-purpose chips — you need specialised chips.6 And to keep up, you need cutting-edge chips.7 AI labs that are stuck with older generations of chips pay more money and spend more time training models.
It’s possible that compute will become less important of an input into AI progress in the future or that much AI training will be done using hardware other than AI chips.8 But it seems very likely that access to cost-effective compute remains vitally important for at least the next five years, and probably beyond that.9
Some ways hardware experts could help positively shape the development of AI include:
- Providing strategic clarity on AI capabilities and progress, in particular the current and future pace and drivers of those things, in order to inform research and decisions relevant to AI governance
- More accurately forecasting progress in the capabilities of AI systems, for example by estimating the doubling time of AI chip cost-effectiveness
- Better understanding how AI chips are used and produced, for example by mapping out the global semiconductor supply chain
- Researching hardware-related governance mechanisms and policies, which seem promising since AI hardware is necessary, quantifiable, physical, and has a concentrated (though global) supply chain.10 (This field is sometimes called “compute governance.”)
- Designing monitoring regimes to make compute usage more transparent, for example by researching a compute monitoring scheme for large AI models
- Determining the feasibility and usefulness of hardware-enabled mechanisms as a tool for AI governance
- Researching ways to limit access to compute to responsible and regulable actors
- Developing prototypes of novel hardware security features
- Understanding how compute governance fits into the broader geopolitical landscape
- Working in government and policy roles on all the above
- This may be some of the most important work to be done with these skills, but there’s less clarity as of this writing about what these roles will look like.
- Doing impactful and safety-oriented work — including liaising with policymakers — from within industry
- Advising policymakers and answering researchers’ questions as an expert, while working on something else, e.g. in industry
- Though this is more speculative, you might work for third-party auditing organisations as part of a future compute governance programme.
There are currently few AI hardware experts working on these areas who are motivated by reducing existential risk that we know of. As of mid-2023, there seem to be about 3–12 existential-risk-focused full-time equivalents (FTEs) forecasting AI progress with a focus on hardware, and about 10–20 such FTEs working on other projects related to compute governance.11
It’s hard to estimate how many additional AI hardware experts are needed, and the answer could change rapidly — we recommend that you do some of your own research on this and talk with people in the field.
There are ways in which this work could end up being net negative. For example, restricting certain actors’ access to compute could increase geopolitical tensions or lead to a concentration of power. Or work on AI hardware could lead to cheaper or more effective chips, accelerating AI progress. Or governance tools like compute monitoring regimes could be exploited by bad actors.
Some compute governance proposals involve monitoring how AI chips are used by companies, which can raise privacy concerns. Finding ways to implement governance while still protecting personal privacy could be valuable work.
If you do take this path, we encourage you to think carefully through the implications of your plans, ideally in collaboration with strategy and policy experts also focused on creating safe and beneficial AI. (See our article about accidental harm and tips on how to avoid it.)
Another potential downside of gaining expertise in AI hardware to reduce catastrophic risks is that roles in industry, where your impact could be ambiguous or even negative, may end up being more appealing in some ways than higher-impact but lower-paid roles in policy or research.
If you believe it might be difficult for you to switch out of high-paying industry positions and move to roles with much more potential to help others and reduce AI risk, you should carefully consider how to mitigate these challenges. You might aim to save more than usual while earning a high salary in case you later end up making less money; you could donate any earnings above a certain threshold; and you can make sure you’re a part of a community that helps you live up to your values.
Want one-on-one advice on pursuing this path?
If you think this path might be a great option for you, but you need help deciding or thinking about what to do next, our team might be able to help.
We can help you compare options, make connections, and possibly even help you find jobs or funding opportunities.
What does working in high-impact AI hardware expert roles actually look like?
Compute-focused AI governance is an exciting, burgeoning field with lots of activity and many open questions to tackle. There are also relevant policy windows open or likely opening soon as public awareness of risks from AI has increased.
The most common kind of role for AI hardware experts is research, though other potentially impactful roles include working as a policymaker or staffer, as a policy analyst,12 or communicating research to policymakers or the public.
Researcher roles are likely to involve things like:
- Investigating hardware-related governance mechanisms or forecasting AI progress, and communicating results from those investigations to decision makers or other researchers
- Interviewing experts on specific topics related to this
- Writing policy briefs
- Advising researchers working on AI governance
- Managing or mentoring others who work on this
Careers in government, especially in the US, may be highly impactful, too. However, it’s not clear yet if the state of policy development on AI has advanced to the point that the government will be aiming to hire AI hardware experts directly. It may at some point become clear to policymakers that AI hardware knowledge is extremely valuable for implementing AI policy, at which point these experts will be in high demand. We have a separate article about opportunities for getting involved in US AI policy.
Some people working in this field today do so for Washington, DC-based think tanks such as:
- Center for a New American Security (CNAS)
- Center for Security and Emerging Technology (CSET)
- Center for Strategic and International Studies (CSIS)
- RAND (in particular the Technology and Security Policy Fellows)
You can also consider research organisations with relatively less of a policy focus, such as:
Careers in industry (including working for chip designers like Nvidia, semiconductor firms, or cloud providers) and academia could be valuable too, though mainly for developing career capital in the form of skills, connections, and credentials, and such work could unintentionally speed up AI progress.
Knowledge in AI hardware could also be used to do grantmaking, field-building, and research or policy work on AI governance topics that aren’t centrally about AI hardware. However, for these paths, the returns to greater AI hardware expertise will likely diminish more steeply.
How to enter AI hardware expert careers
Though it’s possible to pick up some amount of hardware knowledge while working as, say, an AI governance researcher focused on other topics, the kind of expertise that’s most needed is the sort you only get after some years of studying or working with hardware.
- If you already have expertise in AI hardware, you can consider applying to research or policy fellowships or for entry-level roles like research assistant. In some cases, it’s possible to transition from a career in hardware or semiconductors directly into a more senior research or policy role, especially if you have some prior experience with AI governance.
- Though it’s not a career, you can also usefully offer to advise people who are already working on governance and policy questions dealing with AI hardware.
- Some of the highest-impact jobs may be at major AI labs like OpenAI, DeepMind, and Anthropic.
- See the US policy fellowship database for a list of policy fellowships.
- We also have AI safety and policy fellowships on our job board.
- If you have a degree (or have otherwise gained skills) related to AI hardware, but have no professional experience, you can consider building career capital by taking roles in industry or maybe academia. You’re likely to get the most useful experience working on AI hardware directly for companies like Nvidia, but other chip and semiconductor companies seem promising too.
- If you don’t yet have experience or skills related to this, it’s unclear whether AI hardware is the best thing for you to focus on. Perhaps it is if you are especially excited about it or feel that you may be an especially good fit for it.
- Studying computer engineering at the undergraduate level is typically required to work in industry. The coursework requires strong ability in science and maths. You may also want to obtain a master’s degree in the field.
- The state of the art in this field is constantly evolving, so you should expect to continue learning after your formal education ends. Working at the most cutting-edge companies will likely give you the best understanding of technological developments.
- An educational background in computer and AI hardware may be sufficient to offer you significant advantages when starting out a career in AI governance, particularly in Washington, DC. Though you’ll likely want to supplement this technical expertise with some policy-related career capital, such as a prestigious fellowship.
Specific types of knowledge and experience that seem promising include knowledge and experience in AI chip architectures and design, hardware security, cryptography, cybersecurity, semiconductor manufacturing and supply chains, cloud computing, machine learning, and distributed computing. It seems especially valuable to have people who also have knowledge useful for AI governance or policy-making more broadly, though this is not necessary.
Learn more
Top recommendations
- Podcast: Lennart Heim on the compute governance era and what has to come after
- Transformative AI and compute – reading list
- Podcast: Danny Hernandez on forecasting and the drivers of AI progress
Further recommendations
- What does it mean to become an expert in AI Hardware?
- Podcast: Prof Allan Dafoe on trying to prepare the world for the possibility that AI will destabilise global politics
- AI governance needs technical work
- Follow the SemiAnalysis newsletter for updates in the semiconductor industry
- Video and transcript of presentation on introduction to compute governance – a general introduction to compute governance by a leading researcher in the field.
- Advice and resources for getting into technical AI governance
- 12 tentative ideas for US AI policy – a list of policy ideas, including several related to compute governance
- What does it take to catch a Chinchilla? Verifying rules on large-scale neural network training via compute monitoring – a research paper by Yonadav Shavit proposing a compute monitoring scheme
Notes and references
- The full paragraph reads: “The future of integrated electronics is the future of electronics itself. The advantages of integration will bring about a proliferation of electronics, pushing this science into many new areas. Integrated circuits will lead to such wonders as home computers – or at least terminals connected to a central computer – automatic controls for automobiles, and personal portable communications equipment. The electronic wristwatch needs only a display to be feasible today.” Gordon Moore would go on to co-found Intel in 1968.↩
- A transistor can now be less than 10 nanometers wide (if measured by “gate length”), ten thousand times smaller than a hair’s breadth. The foremost example of the complexity of semiconductor manufacturing is ASML’s extreme ultraviolet (EUV) photolithography machines. These machines, which print circuit patterns onto silicon wafers, are the size of living rooms, cost $150 million, and require incredibly advanced components:
- An extreme ultraviolet lithography machine is a technological marvel. A generator ejects 50,000 tiny droplets of molten tin per second. A high-powered laser blasts each droplet twice. The first shapes the tiny tin, so the second can vaporise it into plasma. The plasma emits extreme ultraviolet (EUV) radiation that is focused into a beam and bounced through a series of mirrors. The mirrors are so smooth that if expanded to the size of Germany they would not have a bump higher than a millimetre. Finally, the EUV beam hits a silicon wafer — itself a marvel of materials science — with a precision equivalent to shooting an arrow from Earth to hit an apple placed on the moon. This allows the EUV machine to draw transistors into the wafer with features measuring only five nanometers – approximately the length your fingernail grows in five seconds. This wafer with billions or trillions of transistors is eventually made into computer chips.↩
- This article uses “AI chip” to refer to a semiconductor specialised in performing operations common in AI model training and inference – examples include Google’s Tensor Processing Units (TPUs) and graphical processing units (GPUs) like the Nvidia H100. An “AI accelerator” is a piece of hardware equipment containing an AI chip, like the Nvidia DGX H100 (which contains 8 Nvidia H100 chips). “AI hardware” is a more general term, which refers not only to AI chips and AI accelerators but also other AI-related hardware such as interconnect technology.↩
- There are other inputs, too, like money and talent, but the effects of those are mediated by the other three (compute, data, and ideas). See for example Buchanan’s The AI Triad and What It Means for National Security Strategy (2020).↩
- Besides being crucial for enabling large training runs, AI hardware is also used to cost-effectively deploy AI models (enabling AI labs to generate revenue at lower cost), to generate synthetic data for training future AI models on, and to carry out experiments when doing AI capabilities research.
More speculatively, it’s possible that we will in the future be able to run autonomous AI researchers that would themselves speed up AI research. If so, having access to more compute would allow AI labs to find ideas faster, and to collect or generate more and better data.↩
- Khan (2020): “Because of their unique features, AI chips are tens or even thousands of times faster and more efficient than CPUs for training and inference of AI algorithms. State-of-the-art AI chips are also dramatically more cost-effective than state-of-the-art CPUs as a result of their greater efficiency for AI algorithms. An AI chip a thousand times as efficient as a CPU provides an improvement equivalent to 26 years of Moore’s Law-driven CPU improvements.”↩
- Khan (2020): “Cutting-edge AI systems require not only AI-specific chips, but state-of-the-art AI chips. Older AI chips — with their larger, slower, and more power-hungry transistors — incur huge energy consumption costs that quickly balloon to unaffordable levels. Because of this, using older AI chips today means overall costs and slowdowns at least an order of magnitude greater than for state-of-the-art AI chips. […] In fact, at top AI labs, a large portion of total spending is on AI-related computing. With general-purpose chips like CPUs or even older AI chips, this training would take substantially longer to complete and cost orders of magnitude more, making staying at the research and deployment frontier virtually impossible.”↩
- For example, data could become a more critical bottleneck, or there could be sudden, large improvements to AI algorithms, such that you need relatively little compute to train powerful models.↩
- See, for example, research suggesting that it is possible to predict future AI systems’ performances on various benchmarks if you only know how much compute is used to train them, like Owen (2023). That suggests there is still plenty of opportunity to improve frontier models by using increasing amounts of compute.↩
- Additional reasons for why compute seems like a promising leverage point for AI governance include:
- The massive energy requirements of large compute clusters
- The fact that not only do you need many AI chips to train frontier models, but these also need to be located in the same facilities (in order to allow information to be passed between them quickly).↩
- I got this estimate by asking some people, tallying the people I know of, and applying some uncertainty to the upper bound. These estimates include only people directly working on these things, not, for example, people doing operations for an organisation doing this work, valuable though that is.
Researchers I’ve spoken to say there is a strong need for AI hardware expertise today, though it’s less clear whether that will still be the case five years from now, and it’s also less clear whether there is a need for many or relatively few additional experts. There are aspects of AI hardware and related areas that no one who is working on reducing existential risk is an expert on, and having just a single person knowledgeable about those aspects could bring a lot of value.↩
- A policy analyst is a researcher (and sometimes a staffer) focused on understanding and informing public policy.↩
- Disclosure: The author of this piece, Erich Grunewald, is an employee of IAPS.↩